The overall aim of this project is to produce a detailed profile of the viral dynamics-host interactions of IBV vaccination in chickens to gain major insights into the virus biology and host responses that will aid in developing better vaccines and control strategies. This overall aim will be achieved through the following 4 work packages.
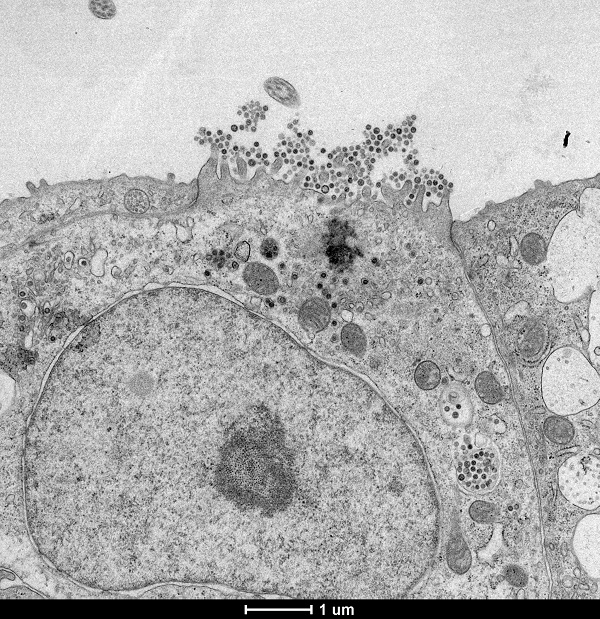
WP 1
Identifying markers of viral attenuation
Using deep learning (DL) to identify patterns of attenuation in existing deep sequencing datasets
We will use a novel supervised deep learning tools to identify patterns in existing deep sequencing viral genome datasets to identify patterns of mutations in either or both disease causing and vaccine viruses. We will use pre-existing serological and molecular data to define labels for machine learning.
Using bioinformatics techniques, we will characterise regions of secondary, tertiary and protein coding structures to identify areas that may play roles in pathogenicity. This will be compared with field data from different pathogenic IBV viruses to explore differences between different viral serotypes.
Anticipated outcomes
WP1 will use DL to identify patterns present in virus genetic material that are shared between attenuated or disease-causing viruses. We will correlate these predictions with bioinformatics analysis characterising location relative to secondary and tertiary structure and genetic distance from known pathogenic IBV isolates.
WP 2
Vaccine virus evolution
2.1 The characterisation of genomic variation post-vaccination and virus vaccine genome stability.
It is thought that as the vaccine virus replicates within the host and will be influenced directly by pressures within that host i.e., immunity. It is therefore important to investigate how these viruses change within infected (or vaccinated) hosts so that we can identify regions of variability in which the virus is prone to change. We will monitor changes in the main IBV immune target ‘S-gene’ to characterise its evolution as the viruses move through the host and over time. We will match this with data from the bird’s immunity. We anticipate that changes observed in both S-gene and the whole genome will help us understand where attenuated viruses are prone to change in vivo. This will provide possible mechanisms by which to determine how these viruses evolve subject to host pressures and how vaccines will perform once inside birds.It is thought that as the vaccine virus replicates within the host and will be influenced directly by pressures within that host i.e., immunity. It is therefore important to investigate how these viruses change within infected (or vaccinated) hosts so that we can identify regions of variability in which the virus is prone to change. We will monitor changes in the main IBV immune target ‘S-gene’ to characterise its evolution as the viruses move through the host and over time. We will match this with data from the bird’s immunity. We anticipate that changes observed in both S-gene and the whole genome will help us understand where attenuated viruses are prone to change in vivo. This will provide possible mechanisms by which to determine how these viruses evolve subject to host pressures and how vaccines will perform once inside birds.
2.2 Identifying dissemination rates of IBV within vaccinated/infected hosts.
It has been shown previously that attenuated vaccine viruses disseminate at a slower rate than pathogenic counterparts within vaccinated hosts. We will investigate host and viral factors as to why attenuated viruses are less able to disseminate through hosts efficiently and evaluate the impact of viral dissemination and changes in the viral genome and how these relate outcomes of infection.
2.3 Predicting host gene expression using Immunofluorescence (IF) slide analysis with deep generative modelling.
The key to an accurate machine learning predictions of gene expression is to generate optimal training material for supervised and unsupervised learning protocols. We will leverage the latest advances in deep learning methodologies to identify/extract high-dimensional features from image data to make predictions informing us regarding viral pathogenicity and host factors.
Anticipated outcomes
Sequence and image data will enable characterisation of viral genetic diversity at different timepoints post-infection/vaccination in host tissues. We will also measure the ability of attenuated viruses to disseminate within infected and vaccinated hosts and correlate differences with identified sequence changes.
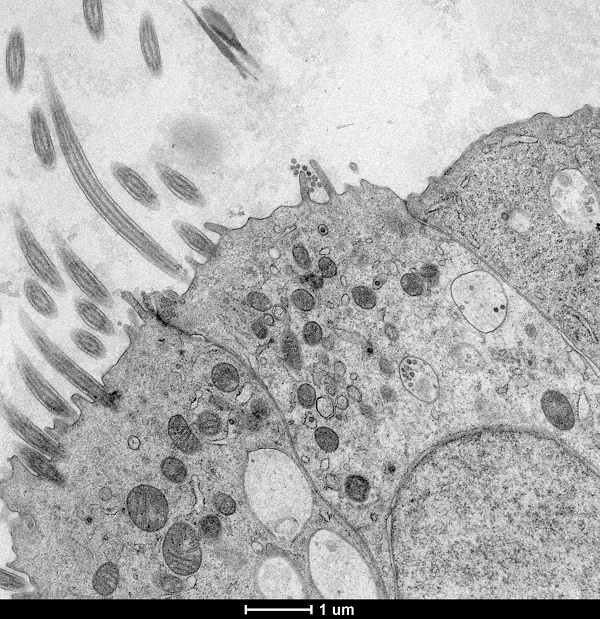
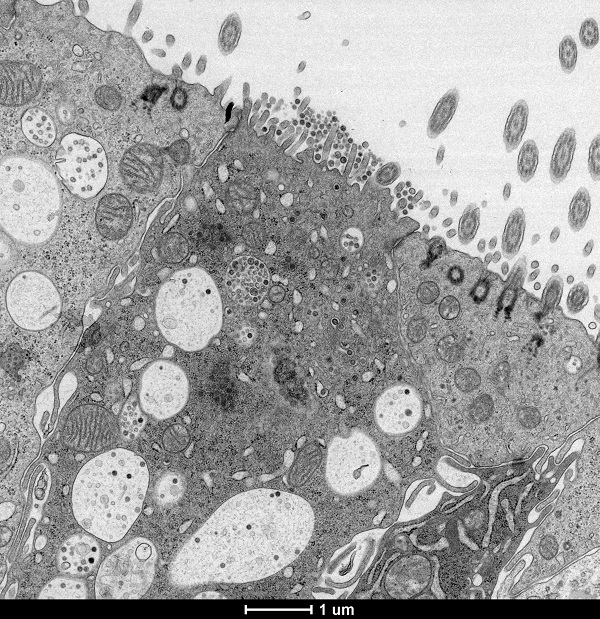
WP 3
Host responses
3.1 Evaluating host gene expression in virus infected tissues to make predictions on virus subtypes based on host transcriptomic response
We will investigate the direct impact of virus upon host gene expression using the 10X Visium Spatial transcriptomics platform. This cutting-edge protocol, combining IF microscopy and Illumina based RNA-Seq, will identify direct overlaps with host cellular gene expression and virus localisation in host tissues. When combined with ultra-deep transcriptomic datasets will create a multi-layered map of spatial gene expression which will help us to understand both the pathological disease process in hosts and the selective forces that drive the evolution of LAVs post-vaccination.
We hypothesise that differences in gene expression between pathogenic/non-disease infected groups will inform about protective correlates of host-pathogen interactions, whilst characterising host cellular pressures upon both pathogenic and attenuated viruses. We will use DL protocols with spatial transcriptomics data to predict labels for different biological processes i.e., pathogenic vs attenuation.
Anticipated outcomes
This objective will classify images based on infection with pathogenic or non-diseases causing viruses using host transcriptomic responses.
WP 4
Combined dataset analysis and validation
By combining the outputs from individual work packages, additional deep learning (DL) will facilitate the accurate identification of molecular signatures of attenuation and pathogenicity
We will combine datasets from the above work packages 1-3 to facilitate DL predictions on markers for pathogenic and non-disease-causing viruses.
Anticipated outcomes
We will combine the different datasets and findings to come up with a detailed profile of the viral dynamics-host interactions of IBV infection in chickens to gain major insights into the how these viruses cause disease, and how hosts respond to the infections, that will aid in developing better vaccines and control strategies.
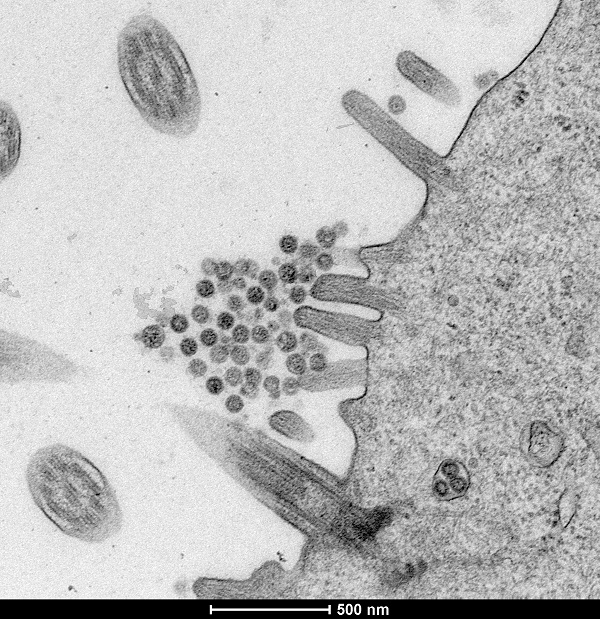